Mean for Population: What It Reveals About Our Society
The United Nations Population Fund (UNFPA) utilizes the concept of mean for population to assess demographic trends globally. Statistical analysis of this mean provides valuable insights for understanding resource allocation and social policy development. Variations in the mean for population, analyzed by researchers at institutions like the Pew Research Center, often correlate with socioeconomic indicators such as access to healthcare and educational opportunities. Therefore, understanding the mean for population allows a deeper comprehension of societal well-being and the impact of various factors on communities.
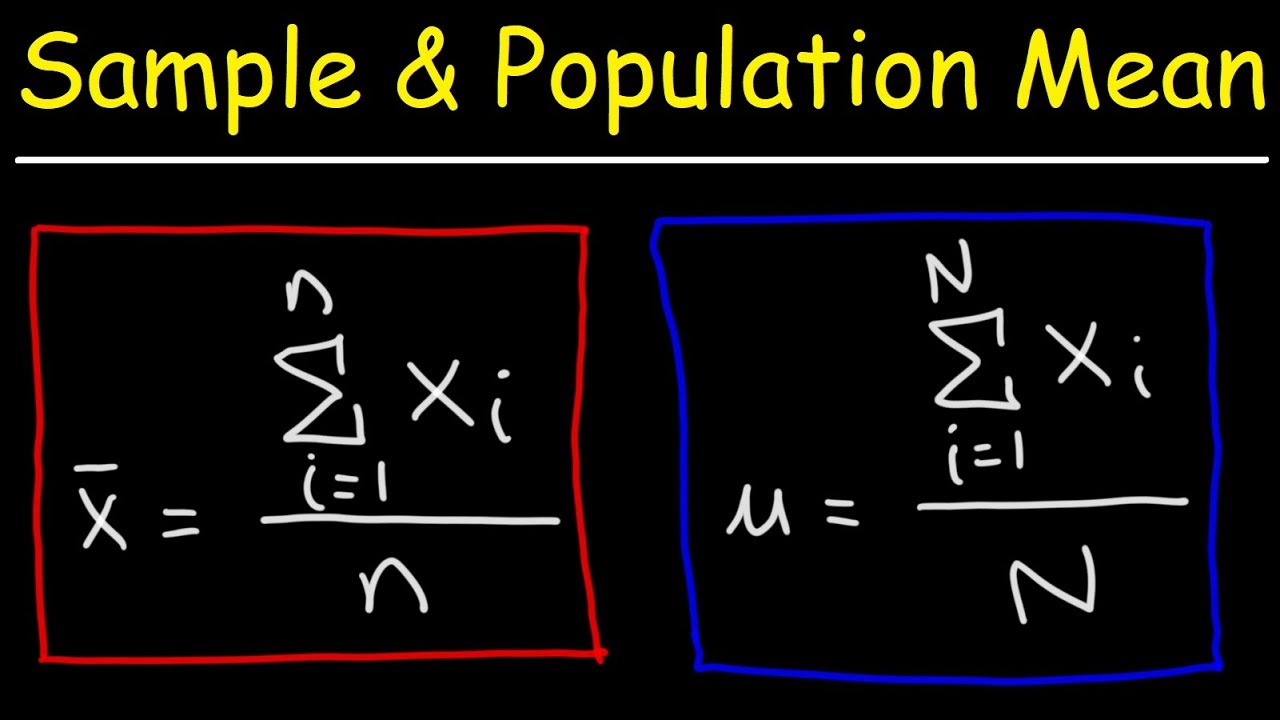
Image taken from the YouTube channel The Organic Chemistry Tutor , from the video titled Sample Mean and Population Mean - Statistics .
In the realm of social sciences, understanding the characteristics of a population is paramount. Statistics provides the tools to dissect complex societal structures, and at the heart of these tools lies the concept of the "mean for population." This is more than just a simple average; it is a potent lens through which we can examine societal well-being, identify pressing challenges, and gauge progress over time.
Defining the "Mean" in a Statistical Context
At its core, the mean, often referred to as the average, is calculated by summing all the values in a dataset and dividing by the number of values.
In statistical terms, it represents the central tendency of a set of numbers. This seemingly simple calculation can unlock profound insights when applied to population data.
The Power of Population Means
The "mean for population" extends this basic concept to an entire group of individuals. When applied across various indicators, it provides a valuable snapshot of the collective experience.
For instance, the mean income of a population can reveal economic disparities, while the mean age at marriage can shed light on evolving social norms.
This aggregate perspective is crucial for understanding overarching trends that might be obscured by individual variations.
Illuminating Trends and Informing Policy
The real power of population means lies in their ability to reveal trends and inform policy decisions.
By tracking changes in the mean over time, researchers and policymakers can identify areas where interventions are needed.
For example, a declining mean score on standardized tests might signal systemic issues within the education system. This signals the need for a policy review. An increasing mean age of retirement may show a need for changes in pension or social security schemes.
Moreover, comparing population means across different regions or demographic groups can highlight inequalities and inform targeted interventions.
Thesis Statement: Exploring Societal Well-being, Challenges, and Progress
This article delves into the significance of population means as indicators of societal health.
By examining various key indicators and their mean values, it will explore how these measures illuminate societal well-being, uncover pressing challenges, and track progress towards a more equitable and prosperous future.
Through careful analysis and critical reflection, we aim to demonstrate the power of the mean as a tool for understanding and improving the lives of individuals and communities around the world.
In observing how population means illuminate trends and inform policy decisions, we begin to appreciate the foundational role that statistical measures play in making sense of large datasets. One measure is particularly important, and it allows us to understand complex societal structures: the mean.
The Power of the Mean: A Central Tendency Measure for Population Data
The mean, as a statistical tool, provides a crucial lens through which to view population data. Understanding its significance and appropriate application is essential for accurate interpretation and informed decision-making. The mean serves as a central tendency measure, a way of summarizing an entire dataset with a single, representative value.
Understanding Central Tendency
Central tendency measures aim to identify the typical or average value within a dataset. While several measures exist, including the median and mode, the mean holds particular importance due to its sensitivity to all values in the data. In essence, the mean provides a balancing point for the data. It's the value around which all other values are distributed. This characteristic makes it valuable when working with population-level data.
Relevant Data Types for Mean Calculation
The mean is most effectively applied to continuous or interval data. These are data types where numerical values have meaningful differences. Some of the most relevant data types for calculating population means include:
- Age: The mean age of a population provides insights into its demographic structure. This is valuable for planning social services and understanding aging trends.
- Income: The mean income reveals the economic well-being of a population. This can highlight income disparities and inform economic policies.
- Education Years: The mean number of years of education reflects a population's human capital. This is important for understanding workforce readiness and educational attainment.
It's important to note that the appropriateness of using the mean depends on the distribution of the data. If the data is heavily skewed by outliers, the mean might not be the best representation of the central tendency.
The Importance of Statistics in Analyzing Population Means
Statistics provides the framework for calculating and interpreting population means. Statistical methods allow us to:
- Estimate population means from samples: Since it's often impractical to collect data from an entire population, we rely on samples. Statistics provides tools to estimate the population mean from a sample mean.
- Assess the uncertainty associated with our estimates: Statistical tests and confidence intervals quantify the margin of error in our estimates. This helps us understand how reliable our conclusions are.
- Compare means across different groups or time periods: Statistical hypothesis testing allows us to determine whether observed differences in means are statistically significant. We can compare differences between populations or see if something changed over time.
Without a solid understanding of statistics, interpreting population means can lead to misleading conclusions.
Demographics and the Mean
The demographics of a population profoundly influence the mean values of various indicators. Demographic factors, such as age structure, gender distribution, and ethnic composition, can create variations in means across different populations or subgroups.
For example, a population with a large proportion of elderly individuals will likely have a higher mean age. Populations with different socioeconomic backgrounds may exhibit different mean income levels. Understanding these relationships is crucial for contextualizing population means and drawing meaningful inferences. Failing to consider demographic factors can lead to inaccurate or incomplete interpretations.
In considering the power of the mean to summarize population-level data, it's natural to ask: What specific insights can we glean by examining the average values of key societal indicators? The following section explores how the mean values of life expectancy, mortality rate, fertility rate, and other social indicators serve as vital signs of societal health and well-being.
Decoding Societal Health: Key Indicators and Their Mean Values
Societal well-being is a multifaceted concept, reflecting a complex interplay of health, economic stability, and social progress. To gain a comprehensive understanding of a population's overall condition, analyzing key indicators and their mean values is essential.
These mean values offer a unique lens through which to view the health and living conditions of various populations, revealing critical insights into their overall well-being.
Life Expectancy: A Mirror to Healthcare and Living Conditions
Mean life expectancy stands as a powerful metric, reflecting the average number of years a newborn is expected to live, assuming mortality patterns remain constant. It acts as a composite indicator, summarizing the collective impact of numerous factors influencing health and longevity.
Unveiling Healthcare and Living Condition Through Life Expectancy
A high mean life expectancy often signifies access to quality healthcare, adequate nutrition, safe living environments, and effective public health programs. Conversely, a lower mean life expectancy can point to deficiencies in these areas.
For instance, regions with limited access to medical care, high rates of infectious diseases, or widespread malnutrition typically exhibit lower mean life expectancies.
Regional Disparities and Contributing Factors
Significant variations in mean life expectancy exist across different regions and countries. Developed nations with robust healthcare systems and high standards of living generally boast higher life expectancies.
Meanwhile, developing countries often grapple with challenges such as poverty, limited access to healthcare, and environmental hazards, resulting in lower mean life expectancies. Factors such as access to clean water, sanitation, and education also play crucial roles.
Mortality Rate and Fertility Rate: Balancing Population Dynamics
Mortality rate, typically expressed as the number of deaths per 1,000 people per year, offers crucial insights into population health and environmental conditions. Similarly, the fertility rate, which represents the average number of children a woman is expected to bear in her lifetime, reflects societal norms, access to family planning, and economic factors.
Interplay of Mortality and Fertility Rates
The relationship between mortality and fertility rates is often complex and interconnected. In populations with high mortality rates, particularly infant and child mortality, fertility rates tend to be higher.
This can be attributed to a desire to ensure the survival of at least some offspring and provide support for aging parents.
Population Health Through Mean Rate Values
The mean values of mortality and fertility rates provide valuable information about population health. A high mean mortality rate can indicate the prevalence of disease, malnutrition, or inadequate healthcare.
Conversely, a low mean mortality rate suggests improved health outcomes and living conditions. High mean fertility rates may strain resources and contribute to rapid population growth, while low mean fertility rates can lead to concerns about aging populations and declining workforces.
Social Indicators: Gauging Societal Well-being
Beyond life expectancy, mortality, and fertility, a range of social indicators provide a more holistic view of societal well-being. These indicators, when examined through their mean values, can reveal important insights into various aspects of a population's life.
Social indicators may include education levels (mean years of schooling), employment rates, poverty rates, access to sanitation, and crime statistics.
For example, the mean years of schooling can reflect the level of human capital within a population, while the mean income can reflect the overall economic prosperity of a society.
By analyzing the mean values of these indicators, policymakers and researchers can gain a deeper understanding of the challenges and opportunities facing different populations.
Census and Sample: Foundation for Calculating Population Means
The accuracy and reliability of population means heavily rely on the quality of the underlying data. Censuses and sample surveys are indispensable tools for collecting the necessary data to calculate these means.
A census aims to enumerate the entire population of a given area, providing a comprehensive snapshot of its demographic characteristics, socioeconomic status, and living conditions. While censuses offer the most accurate representation of a population, they are often expensive and time-consuming to conduct.
Sample surveys, on the other hand, involve collecting data from a representative subset of the population. These surveys can be more cost-effective and efficient than censuses, but they require careful design and implementation to ensure that the sample accurately reflects the characteristics of the larger population. The sample size has to be representative and large enough.
Both censuses and sample surveys play crucial roles in providing the data needed to calculate meaningful population means.
Driving Forces: Factors Shaping the Mean for Population
Understanding the mean for population requires acknowledging the complex web of factors that influence it. These forces operate at various levels, from individual socioeconomic circumstances to global phenomena, shaping the average values we observe across different societal indicators.
Socioeconomic Factors: The Foundation of Population Means
Socioeconomic conditions are arguably the most fundamental drivers of population means. Poverty, for instance, exerts a profound impact on life expectancy, mortality rates, and even fertility rates. Limited access to nutritious food, healthcare, and safe living environments directly undermines health outcomes.
Similarly, inequality within a population can skew the mean, masking the struggles of marginalized groups. A high mean income, for example, might conceal the fact that a significant portion of the population lives below the poverty line. It's essential to consider income distribution, not just the average, for a more accurate portrayal of societal well-being.
Government Policies and Interventions: Steering the Course
Government policies and interventions play a critical role in shaping population means. Public health initiatives, such as vaccination programs and sanitation projects, can dramatically improve life expectancy and reduce mortality rates, thus impacting the mean value of these indicators.
Educational policies, particularly those focused on girls' education, have been shown to correlate with lower fertility rates and improved child health outcomes. Effective social safety nets, including unemployment benefits and food assistance programs, can mitigate the negative impacts of poverty on population health.
Global Factors: A World of Influence
Global trends also exert a significant influence on population means. Globalization, with its interconnectedness and exchange of goods, ideas, and technologies, can lead to both improvements and challenges. While access to new medicines and technologies can improve health outcomes, increased exposure to unhealthy diets and lifestyles can contribute to chronic diseases.
Climate change is an increasingly pressing concern, posing a direct threat to population health. Extreme weather events, food shortages, and the spread of infectious diseases can all negatively impact life expectancy and mortality rates, affecting the mean values of these indicators on a population level.
The United Nations: A Global Observatory
The United Nations (UN) plays a pivotal role in analyzing population data and trends worldwide. Through organizations like the United Nations Population Fund (UNFPA) and the World Health Organization (WHO), the UN collects, analyzes, and disseminates data on a wide range of population indicators.
These data are used to inform policy decisions at the national and international levels, helping governments and organizations to address challenges related to poverty, health, and sustainable development. The UN's analyses often highlight disparities across regions and populations, calling attention to areas where interventions are most needed. Their standardized methodologies and global reach make them essential for understanding population dynamics.
From Data to Action: Applications of Mean for Population in Policy and Planning
Having explored the factors that shape population means, it's crucial to understand how this data translates into tangible action. Governments and organizations worldwide leverage the mean for population as a compass, guiding policy decisions, resource allocation, and development strategies. The effective application of this statistical tool can lead to improved societal outcomes and a more equitable distribution of resources.
Guiding Policy Decisions with Population Averages
Governments routinely use the mean for population to inform policy-making across various sectors. For example, mean income data guides taxation policies and social welfare programs. Understanding the average income levels within different demographics allows policymakers to design targeted interventions that address income inequality and poverty.
Mean educational attainment levels inform education policies. By analyzing the average years of schooling completed within a population, governments can identify areas where educational resources need to be strengthened. This data can support initiatives aimed at improving literacy rates, increasing access to higher education, and promoting lifelong learning.
Healthcare policy heavily relies on mean life expectancy and mortality rates. These indicators help governments assess the effectiveness of their healthcare systems and identify areas where improvements are needed. Resource allocation, preventive care programs, and public health campaigns are often shaped by these population averages.
Successful Interventions Informed by the Mean
Numerous successful interventions have been driven by a thorough understanding of the mean for population. For instance, targeted interventions aimed at reducing infant mortality rates in specific regions have been informed by analyzing the mean infant mortality rates in those areas.
Similarly, programs designed to combat malnutrition in developing countries often rely on data regarding the mean nutritional intake of children. These programs provide nutritional support, education on healthy eating habits, and access to fortified foods.
Another illustration can be seen in interventions addressing gender inequality in education. Understanding the mean years of schooling for girls versus boys in specific communities has driven initiatives to promote girls' education through scholarships, mentorship programs, and community awareness campaigns. These interventions demonstrate the power of using population means to identify and address specific societal challenges.
Resource Allocation and Development Planning
The mean for population plays a pivotal role in resource allocation and development planning. Governments and organizations use population averages to determine how to distribute resources effectively across different regions and sectors.
For instance, funding for infrastructure projects, such as roads, schools, and hospitals, is often allocated based on the mean population density and the average distance to essential services in a given area.
Development planning also relies on understanding the mean age of the population. A population with a high mean age may require increased investment in healthcare services for the elderly, while a population with a low mean age may require more resources for education and childcare.
Furthermore, data on mean unemployment rates informs the development of job creation programs and skills training initiatives. By analyzing the average unemployment rates across different demographics, policymakers can design targeted interventions to address unemployment and promote economic growth.
In conclusion, the mean for population serves as a powerful tool for informing policy-making, guiding resource allocation, and driving development planning. By effectively utilizing population averages, governments and organizations can design targeted interventions that address societal challenges, promote equity, and improve overall well-being.
Beyond the Average: Addressing Limitations and Considering Alternative Measures
While the mean provides a valuable snapshot of population trends, it's essential to acknowledge its limitations and consider alternative statistical measures for a more nuanced understanding of societal realities. Over-reliance on the mean can obscure significant disparities within a population and render the impact of outliers.
The Masking Effect: How the Mean Can Hide Disparities
The mean, by its very nature, averages values across an entire group. This process can conceal substantial inequalities. For example, a high mean income in a city might mask the fact that a large segment of the population lives in poverty while a small percentage enjoys extreme wealth.
Similarly, a country with a high mean life expectancy may still have pockets of vulnerable populations with significantly lower life spans due to factors like lack of access to healthcare or poor living conditions.
Therefore, solely relying on the mean to assess societal well-being paints an incomplete, and potentially misleading, picture. It's crucial to delve deeper and examine the distribution of data to uncover hidden disparities.
The Outlier Problem: The Mean's Susceptibility to Extreme Values
Outliers, or extreme values, can significantly skew the mean, misrepresenting the typical experience within a population. A single billionaire, for example, can disproportionately inflate the mean income of a small town.
Similarly, in educational attainment, a few individuals with advanced degrees can raise the average years of schooling completed, even if many residents have not finished high school.
The mean's sensitivity to outliers necessitates caution when interpreting the data, especially in populations with known income or educational disparities.
Alternative Statistical Measures: Providing a More Comprehensive View
To overcome the limitations of the mean, it's crucial to consider other statistical measures that offer a more comprehensive understanding of the population.
The Median: A Robust Measure of Central Tendency
The median, the middle value in a dataset, is less susceptible to outliers than the mean. In the context of income, the median income provides a more accurate representation of the typical income level, as it is not significantly affected by extreme wealth. Similarly, the median age offers a more stable measure of the population's central age.
Standard Deviation: Gauging Variability
Standard deviation measures the spread or variability of data around the mean. A high standard deviation indicates that data points are widely dispersed, suggesting significant heterogeneity within the population. For instance, a high standard deviation in income levels would confirm significant income inequality.
Other Measures
Other important measures include: mode, range, interquartile range, and percentiles, all of which provide additional insights into the distribution and characteristics of the population.
By considering these alternative measures, a more detailed and nuanced picture of the population emerges, overcoming the limitations of relying solely on the mean.
Sample Limitations: Representing the Whole Population
In many cases, collecting data from the entire population is impractical or impossible. Therefore, researchers often rely on samples to estimate population means. However, samples can introduce limitations that must be carefully considered.
Sample Size and Representativeness
A small sample size may not accurately represent the diversity and characteristics of the entire population. Similarly, if the sample is not randomly selected or is biased towards a particular subgroup, the results may not be generalizable to the whole population. Ensuring an adequate sample size and employing appropriate sampling techniques are crucial for minimizing bias and maximizing the representativeness of the sample.
Margin of Error: Acknowledging Uncertainty
Margin of error quantifies the uncertainty associated with sample estimates. It provides a range within which the true population mean is likely to fall. A smaller margin of error indicates greater precision. When interpreting sample data, it is essential to consider the margin of error and acknowledge the inherent uncertainty in the estimates.
Stratification
Stratification may be used to improve the accuracy of the mean, by proportionally representing subgroups of the population.
By acknowledging the limitations of samples and carefully considering factors like sample size, representativeness, and margin of error, researchers can draw more reliable inferences about the population mean.
Video: Mean for Population: What It Reveals About Our Society
FAQs: Understanding Population Mean
What does the "mean for population" actually represent?
The mean for population, in simple terms, is the average value you get when you sum up a particular characteristic for every individual in a population and then divide by the total number of individuals. This single number provides a central tendency for the entire group.
How can the mean for population reveal insights about society?
Analyzing the mean for population, when considering factors like income, education level, or even health metrics, can highlight societal trends. Significant disparities in these averages across different demographic groups, for example, may expose inequalities and systemic issues.
What are the limitations of relying solely on the mean for population to understand society?
While informative, the mean for population only offers a snapshot. It doesn't show the distribution of values within the population. Extreme outliers or significant variations within subgroups might be masked when only looking at the average.
Why is it important to consider sample size when interpreting the mean for population?
The accuracy and reliability of a calculated mean for population significantly depend on the sample size used. Larger, more representative samples provide a more robust estimation and minimize the potential for biased or misleading conclusions.