Social Implications: AI Mental Health - Risks?
The intersection of artificial intelligence and mental healthcare presents a multifaceted array of social implications, demanding careful scrutiny from ethicists, policymakers, and the public. Algorithmic bias, a prevalent concern in AI development, manifests in mental health applications through skewed datasets and discriminatory outputs, affecting diagnosis and treatment recommendations for various demographic groups. The American Psychiatric Association, a leading professional organization, emphasizes the necessity of integrating ethical guidelines into the development and deployment of AI-driven mental health tools to mitigate potential harm. Concurrently, the increasing reliance on AI-powered chatbots, such as Woebot, for therapeutic interventions raises critical questions about data privacy and the potential erosion of human empathy in clinical practice. Furthermore, the accessibility divide related to digital literacy and technological infrastructure exacerbates existing inequalities, potentially leaving vulnerable populations behind in the AI-driven transformation of mental healthcare, highlighting the significant social implications.
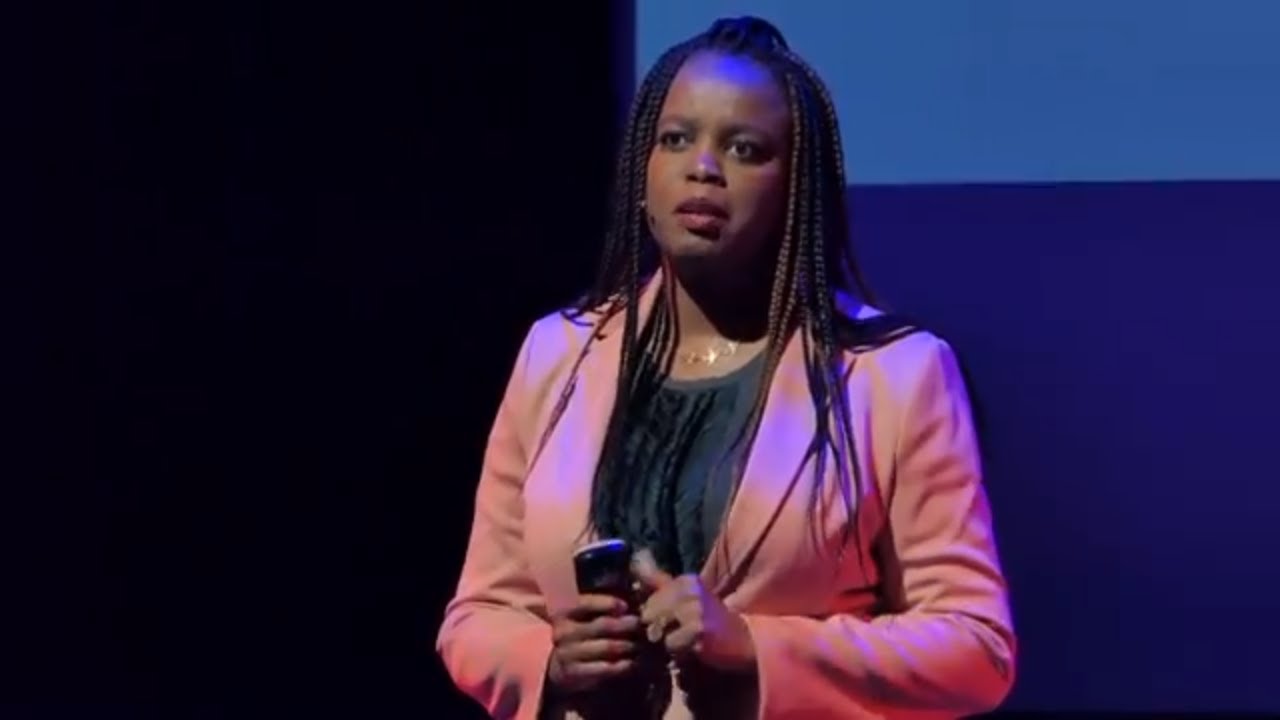
Image taken from the YouTube channel TEDx Talks , from the video titled The 5 Principles of Social Impact | Marian Spier | TEDxErasmusUniversityRotterdam .
AI's Transformative Role in Mental Healthcare: Navigating the Ethical Landscape
Artificial intelligence (AI) is rapidly transforming numerous sectors, and mental healthcare is no exception. From AI-powered chatbots offering immediate support to sophisticated diagnostic tools capable of identifying subtle indicators of mental distress, the integration of AI promises to revolutionize how we approach mental well-being. Furthermore, AI holds the potential to personalize treatment plans, tailoring interventions to the unique needs of each individual.
However, this technological revolution brings with it a complex web of ethical and societal implications that demand careful consideration. As AI systems become increasingly integrated into mental healthcare, it is crucial to critically examine the potential risks and benefits.
The Rise of AI in Mental Healthcare
The application of AI in mental healthcare is multifaceted, spanning various critical areas:
-
AI Chatbots: These conversational agents offer immediate, accessible support, providing a listening ear and guidance for individuals experiencing emotional distress.
-
Diagnostic Tools: AI algorithms can analyze vast datasets to identify patterns and predict the likelihood of mental health conditions, potentially enabling earlier intervention.
-
Personalized Treatment: AI can tailor treatment plans based on individual patient data, optimizing the effectiveness of interventions.
These are just a few examples of how AI is reshaping the mental healthcare landscape. As AI technology continues to evolve, its potential applications in this field will undoubtedly expand.
The Imperative for Ethical Scrutiny
The increasing reliance on AI in mental healthcare necessitates a rigorous examination of its ethical and societal implications. Unfettered integration without adequate safeguards could exacerbate existing inequalities and introduce new forms of harm.
Several compelling reasons underscore the importance of this ethical scrutiny:
-
Protecting Vulnerable Populations: Mental health conditions can significantly impair an individual's capacity to advocate for themselves. Careful oversight is required to ensure that AI systems do not exploit these vulnerabilities.
-
Ensuring Data Privacy: Mental health data is incredibly sensitive, and breaches of privacy could have devastating consequences. Robust data protection measures are essential to safeguard patient information.
-
Addressing Algorithmic Bias: AI algorithms can perpetuate and amplify existing societal biases, leading to discriminatory outcomes in mental healthcare. Fairness-aware AI development is crucial to mitigate this risk.
-
Maintaining Human Connection: Mental healthcare relies heavily on empathy and the therapeutic relationship. It is imperative to ensure that AI systems complement, rather than replace, human interaction.
Scope, Objectives, and the Need for a Multidisciplinary Approach
This analysis aims to provide a comprehensive overview of the key ethical challenges arising from the integration of AI in mental healthcare. Our scope encompasses a wide range of issues, including algorithmic bias, data privacy, informed consent, transparency, accessibility, autonomy, dehumanization, stigma, responsibility, and commodification.
Our objectives are to:
- Identify the potential risks and benefits of AI in mental healthcare.
- Examine the ethical dilemmas that arise from its deployment.
- Propose recommendations for responsible AI implementation.
- Highlight the need for collaboration among stakeholders.
Addressing these complex ethical issues requires a multidisciplinary approach. Collaboration between psychiatrists, AI developers, ethicists, policymakers, and patients is essential to ensure that AI is used responsibly and ethically in mental healthcare. This collective effort can help shape a future where AI enhances, rather than harms, mental well-being.
Algorithmic Bias: Ensuring Equitable Mental Health Support
AI's transformative potential in mental healthcare is undeniable. However, the promise of improved diagnostics and personalized treatment hinges on addressing a critical challenge: algorithmic bias. If left unchecked, these biases can exacerbate existing inequalities, hindering equitable access to and quality of mental health support, particularly for vulnerable populations.
Defining and Identifying Algorithmic Bias
Algorithmic bias arises when AI models systematically produce unfair or discriminatory outcomes. This typically stems from biased data used during the model's training phase, reflecting societal prejudices or skewed representations of certain demographic groups.
The manifestations of algorithmic bias are diverse. They can appear in the form of:
- Sampling bias, where the training data does not accurately represent the population the model is intended to serve.
- Labeling bias, where the labels assigned to data points reflect subjective opinions or prejudices.
- Measurement bias, where the features used to train the model are not equally accurate or relevant across different groups.
Bias can also emerge from the algorithms themselves, especially if they are designed to optimize for specific outcomes without considering fairness metrics. It is crucial to recognize that AI is not inherently neutral. It is a reflection of the data and the perspectives used to create it.
The Impact of Bias on Mental Healthcare Equity
The implications of algorithmic bias for mental healthcare equity are profound. AI systems used for diagnosis, treatment planning, or risk assessment may systematically disadvantage certain groups, leading to misdiagnosis, inappropriate treatment recommendations, and denial of access to necessary care.
For example, if an AI diagnostic tool is primarily trained on data from predominantly white populations, it may be less accurate in identifying mental health conditions in individuals from other racial or ethnic backgrounds. Similarly, an AI-powered chatbot designed to provide support may inadvertently perpetuate harmful stereotypes or cultural insensitivity, leading to negative outcomes for marginalized communities.
These biases can reinforce existing disparities in mental healthcare, making it even more difficult for vulnerable populations to receive the support they need. The consequences can be particularly severe for individuals facing multiple forms of marginalization, such as those from racial or ethnic minorities who also identify as LGBTQ+ or have disabilities. The use of biased algorithms can create a cycle of inequity, further entrenching these systemic problems.
Strategies for Mitigating Algorithmic Bias
Addressing algorithmic bias requires a multi-faceted approach that spans data collection, algorithm design, and ongoing monitoring. It is not a one-time fix but an ongoing process of critical evaluation and refinement.
-
Diverse and Representative Datasets: The foundation of any fair AI system is a diverse and representative dataset. This means actively seeking out and including data from underrepresented populations, ensuring that the training data accurately reflects the diversity of the population the model will serve. Data augmentation techniques can also be used to increase the representation of minority groups.
-
Fairness-Aware Algorithms: Develop and employ algorithms that are specifically designed to minimize bias. These algorithms incorporate fairness metrics into their objective functions, ensuring that the model's predictions are equitable across different groups. Techniques such as adversarial debiasing and re-weighting can be used to mitigate bias during the training process.
-
Transparency and Explainability: Promote transparency in AI decision-making by using interpretable AI models. These models allow users to understand how the AI system arrived at a particular prediction, making it easier to identify and correct potential biases. Explainable AI (XAI) techniques can provide insights into the factors influencing the model's decisions.
-
Ongoing Monitoring and Evaluation: Continuously monitor and evaluate AI systems for bias after deployment. This involves tracking key fairness metrics and regularly assessing the model's performance across different demographic groups. Feedback from users and stakeholders should be actively solicited and incorporated into the evaluation process.
-
Ethical Oversight and Collaboration: Establish ethical oversight boards to review AI development and deployment in mental healthcare. These boards should include experts from diverse fields, including ethics, law, computer science, and mental health, as well as representatives from affected communities. Collaboration between AI developers, clinicians, and ethicists is essential to ensure that AI systems are developed and used responsibly.
By implementing these strategies, we can harness the power of AI to promote equitable access to and quality of mental health support for all. Failing to address algorithmic bias undermines the potential benefits of AI and perpetuates harmful disparities in healthcare.
Data Privacy: Protecting Sensitive Mental Health Information
AI’s transformative potential in mental healthcare is undeniable. However, alongside the promise of improved diagnostics and personalized treatment lies a crucial responsibility: safeguarding the privacy of sensitive mental health information. The collection, storage, and utilization of this data in AI systems introduces significant risks that demand careful consideration and robust protective measures.
The Vulnerability of Mental Health Data
Mental health data is inherently sensitive. It can include diagnoses, treatment histories, therapy session notes, medication records, and even personal thoughts and feelings shared through AI-powered chatbots. The unauthorized access or disclosure of this information can have devastating consequences for individuals.
These consequences include:
-
Social stigma and discrimination: Disclosure of mental health conditions can lead to prejudice in employment, housing, and social relationships.
-
Emotional distress and psychological harm: Breaches of privacy can trigger anxiety, depression, and a loss of trust in healthcare providers.
-
Financial exploitation: Mental health information can be used to target vulnerable individuals for scams or discriminatory insurance practices.
-
Legal ramifications: In some cases, unauthorized disclosure may violate privacy laws and result in legal action.
The aggregation of mental health data from multiple sources further amplifies these risks. AI algorithms can analyze these large datasets to identify patterns and predict future behavior. However, this capability also raises concerns about profiling and the potential for misuse of personal information.
Navigating the Regulatory Landscape: GDPR and HIPAA
Protecting mental health data requires strict adherence to relevant data protection regulations. Two of the most prominent are the General Data Protection Regulation (GDPR) and the Health Insurance Portability and Accountability Act (HIPAA).
GDPR Compliance
The GDPR, applicable in the European Union, imposes stringent requirements on the processing of personal data, including mental health information. It mandates that data processing be lawful, fair, and transparent, and that individuals have the right to access, rectify, and erase their data.
Specifically, organizations must:
-
Obtain explicit consent from individuals before collecting and processing their mental health data.
-
Implement appropriate technical and organizational measures to protect data from unauthorized access or disclosure.
-
Conduct data protection impact assessments (DPIAs) to identify and mitigate privacy risks.
-
Notify data protection authorities and affected individuals in the event of a data breach.
HIPAA Compliance
HIPAA, in the United States, focuses on protecting the privacy and security of protected health information (PHI), including mental health data. It establishes standards for the use and disclosure of PHI, as well as requirements for data security and breach notification.
Under HIPAA, covered entities (e.g., healthcare providers, health plans) must:
-
Implement administrative, physical, and technical safeguards to protect PHI.
-
Provide individuals with access to their health information and the right to request amendments.
-
Obtain authorization before using or disclosing PHI for purposes other than treatment, payment, or healthcare operations.
-
Comply with the HIPAA Privacy Rule, Security Rule, and Breach Notification Rule.
Anonymization and Pseudonymization: Techniques for Enhancing Privacy
While regulatory compliance is essential, it is not always sufficient to guarantee data privacy. Anonymization and pseudonymization are techniques that can further reduce the risk of identifying individuals from their mental health data.
Anonymization
Anonymization involves removing or altering data elements in such a way that it is impossible to re-identify the individuals to whom the data relates. This typically requires removing direct identifiers (e.g., names, addresses, phone numbers) and indirect identifiers (e.g., dates of birth, zip codes) that, when combined, could lead to re-identification.
Successfully anonymizing mental health data can be challenging. Given the sensitive and personal nature of the information, even seemingly innocuous data points can potentially be linked to individuals. Therefore, robust anonymization techniques, such as data masking, generalization, and suppression, are often necessary.
Pseudonymization
Pseudonymization involves replacing direct identifiers with pseudonyms, such as codes or tokens. This makes it more difficult to identify individuals directly from the data, but it does not eliminate the possibility of re-identification.
Unlike anonymization, pseudonymization allows for the re-linking of data to individuals if the pseudonymization key is available. This can be useful for research purposes or for providing personalized treatment while maintaining a degree of privacy.
However, it is crucial to protect the pseudonymization key from unauthorized access. If the key is compromised, the data can be easily re-identified, negating the privacy benefits of pseudonymization.
Ultimately, protecting sensitive mental health information in AI systems requires a multi-faceted approach. This includes complying with data protection regulations, implementing robust anonymization and pseudonymization techniques, and fostering a culture of privacy awareness among developers, clinicians, and patients. Only through such concerted efforts can we harness the power of AI to improve mental healthcare while upholding the fundamental right to privacy.
Informed Consent: Transparency and Autonomy in AI-Driven Treatment
AI’s transformative potential in mental healthcare is undeniable. However, alongside the promise of improved diagnostics and personalized treatment lies a crucial responsibility: safeguarding the privacy of sensitive mental health information. This imperative extends to the equally critical domain of informed consent, particularly when AI systems are integrated into the therapeutic process. Achieving genuine informed consent in the context of AI-driven interventions presents unique challenges that demand careful consideration and proactive solutions.
The Multifaceted Challenges of Informed Consent in the AI Era
Obtaining truly informed consent is a cornerstone of ethical medical practice. It requires patients to understand the nature of their treatment, its potential benefits and risks, and any available alternatives.
The introduction of AI into mental healthcare significantly complicates this process.
Comprehension Barriers: AI's Complexity and Patient Understanding
One primary challenge lies in the complexity of AI algorithms themselves. Many patients may lack the technical background to fully grasp how an AI system arrives at its recommendations or how it processes their data. This lack of understanding can undermine their ability to make truly informed decisions about their care.
Furthermore, vulnerable populations, such as those with cognitive impairments or limited literacy, may face even greater barriers to comprehending the intricacies of AI-driven treatment.
Power Dynamics and Coercion Risks
The inherent power imbalance between healthcare providers and patients can also be exacerbated by the use of AI. Patients may feel pressured to accept AI-driven recommendations, particularly if they perceive the technology as infallible or if their clinicians strongly advocate for its use. This risk of coercion is especially concerning when dealing with individuals experiencing acute mental health crises or those who are heavily reliant on their caregivers.
The Imperative of Transparency in AI-Augmented Therapy
Transparency is paramount to upholding patient autonomy in the age of AI.
Disclosing AI Involvement: A Foundational Requirement
Patients have a right to know when AI is being used in their treatment, the specific purpose of the AI system, and how it contributes to the overall care plan. This disclosure should be clear, concise, and easily understandable, avoiding technical jargon whenever possible.
Explaining AI's Role: Benefits, Limitations, and Potential Biases
Beyond simply disclosing AI involvement, clinicians must also explain the potential benefits and limitations of the technology. This includes outlining the types of decisions the AI system is capable of making, the data it relies on, and any potential biases that may affect its recommendations.
Critically, patients must be made aware that AI is a tool to augment, not replace, human clinical judgment.
Providing Access to Information: Empowering Patient Agency
Transparency also entails providing patients with access to relevant information about the AI system, such as its algorithms, data sources, and performance metrics. While complete transparency may not always be feasible due to proprietary concerns, efforts should be made to provide as much information as possible without compromising intellectual property rights.
Legal and Ethical Foundations of Consent in AI Interventions
The integration of AI into mental healthcare necessitates a re-evaluation of existing legal and ethical standards for informed consent.
Adherence to Established Principles: Autonomy, Beneficence, and Non-Maleficence
The fundamental principles of autonomy, beneficence, and non-maleficence remain central to ethical decision-making in AI-driven treatment. Patients must be empowered to make their own choices, and healthcare providers must strive to maximize benefits while minimizing potential harm.
Compliance with Data Protection Regulations: GDPR and HIPAA
Adherence to data protection regulations such as GDPR and HIPAA is crucial to safeguarding patient privacy and ensuring the ethical use of AI in mental healthcare. These regulations mandate that patients have the right to access, correct, and delete their data, and that data is used only for purposes to which they have explicitly consented.
Establishing Clear Guidelines and Protocols: Ensuring Accountability
Healthcare institutions and professional organizations should develop clear guidelines and protocols for obtaining informed consent in AI-driven mental healthcare. These guidelines should address issues such as transparency, comprehension, voluntariness, and the right to withdraw consent at any time. Moreover, they should establish clear lines of responsibility for ensuring that these standards are met.
Informed Consent: Transparency and Autonomy in AI-Driven Treatment AI’s transformative potential in mental healthcare is undeniable. However, alongside the promise of improved diagnostics and personalized treatment lies a crucial responsibility: safeguarding the privacy of sensitive mental health information. This imperative extends to the equally critical need for transparency and explainability in AI's decision-making processes.
Transparency and Explainability: Unveiling the "Black Box" of AI Decision-Making
The integration of Artificial Intelligence (AI) into mental healthcare presents unprecedented opportunities. However, it also introduces a significant challenge: the "black box" problem. This refers to the opaque nature of many AI systems, particularly complex machine learning models, where the reasoning behind their decisions is often obscure and difficult to understand. Addressing this issue is crucial for building trust, ensuring accountability, and ultimately, providing ethical and effective mental healthcare.
Understanding the "Black Box" in AI
The "black box" problem arises from the intricate algorithms and vast datasets that power many AI systems. While these systems can achieve impressive accuracy in tasks such as diagnosis and treatment prediction, their internal workings are often hidden from view.
This lack of transparency can be problematic in mental healthcare, where understanding the why behind a recommendation or decision is as important as the what.
For instance, if an AI system suggests a particular treatment plan, clinicians and patients need to understand the factors that led to this recommendation. Without this understanding, it becomes difficult to assess the validity of the suggestion, identify potential biases, or justify the decision to the patient.
The Imperative for Interpretable AI Models
To overcome the "black box" problem, there is a growing emphasis on developing and utilizing interpretable AI models.
Interpretable AI aims to create systems whose decision-making processes are transparent and understandable to humans. This can be achieved through various techniques, such as using simpler models, visualizing decision paths, or providing explanations for individual predictions.
The benefits of interpretable AI in mental healthcare are manifold:
- Enhanced Trust: When clinicians and patients can understand how an AI system arrives at a particular conclusion, they are more likely to trust its recommendations.
- Improved Accuracy: By understanding the factors that influence an AI's decisions, clinicians can identify potential errors or biases in the system and take corrective action.
- Increased Accountability: Transparency in AI decision-making makes it easier to assign responsibility when things go wrong. If an AI system makes an incorrect diagnosis or recommends an inappropriate treatment, it is important to be able to trace the decision back to its source and determine who is accountable.
- Ethical Considerations: The principle of beneficence, or doing good, requires that AI systems used in healthcare are safe and effective. Transparency is essential for ensuring that these systems meet this ethical obligation.
Methods for Enhancing Transparency: Explainable AI (XAI)
Explainable AI (XAI) encompasses a range of techniques aimed at making AI systems more transparent and understandable. These techniques can be broadly categorized into:
-
Model-Agnostic Methods: These methods can be applied to any AI model, regardless of its complexity. Examples include:
- Feature Importance: Identifying the features that have the greatest influence on the model's predictions.
- Partial Dependence Plots: Visualizing the relationship between a feature and the model's output.
- SHAP (SHapley Additive exPlanations) Values: Assigning a value to each feature that represents its contribution to the prediction.
- Model-Specific Methods: These methods are tailored to specific types of AI models. For example, decision tree models are inherently interpretable because their decision-making process can be easily visualized as a series of branching rules.
- Rule-Based Systems: In these systems, decisions are based on predefined rules that are explicitly defined and easily understood.
- Natural Language Explanations: Providing explanations of the AI's reasoning in plain language that is accessible to clinicians and patients.
Challenges in Implementing XAI
While XAI holds great promise, its implementation in mental healthcare faces several challenges:
- Complexity of Mental Health Data: Mental health data is often complex, heterogeneous, and subjective. This can make it difficult to develop interpretable AI models that can accurately capture the nuances of mental illness.
- Need for Clinician Expertise: Interpreting the explanations provided by XAI systems requires clinical expertise. Clinicians need to be able to critically evaluate the AI's reasoning and determine whether it is consistent with their own clinical judgment.
- Balancing Accuracy and Interpretability: There is often a trade-off between the accuracy and interpretability of AI models. More complex models may achieve higher accuracy, but they are also more difficult to understand.
- Data Privacy: XAI methods may inadvertently reveal sensitive information about patients. It is important to carefully consider data privacy when implementing XAI techniques.
Addressing the "black box" problem is not merely a technical challenge; it is an ethical imperative. By embracing interpretable AI models and implementing robust XAI techniques, we can ensure that AI systems in mental healthcare are transparent, accountable, and ultimately, beneficial to patients. The path forward requires interdisciplinary collaboration, thoughtful policy development, and a commitment to prioritizing human understanding in the age of artificial intelligence.
Accessibility and Equity: Bridging the Gap in Mental Healthcare Access with AI
AI’s transformative potential in mental healthcare is undeniable. However, alongside the promise of improved diagnostics and personalized treatment lies a crucial responsibility: ensuring equitable access to these advancements. The question remains: do AI tools exacerbate existing disparities, or can they genuinely bridge the gap in mental healthcare access, particularly for underserved populations?
AI: A Double-Edged Sword for Access
The introduction of AI in mental healthcare presents a complex duality. On one hand, AI-powered tools offer the potential to overcome geographical barriers, reduce costs, and provide immediate support to individuals who may otherwise face long waiting lists or lack of access to specialists.
Teletherapy platforms driven by AI, for instance, can connect patients in remote areas with therapists regardless of location. Similarly, AI chatbots can offer preliminary assessments and guidance, acting as a first point of contact for individuals hesitant to seek traditional care.
However, this optimistic outlook is tempered by the risk of further marginalizing vulnerable communities. The digital divide, characterized by unequal access to technology and internet connectivity, could exclude those most in need from benefiting from AI-driven solutions.
Furthermore, the cost of these technologies, even if lower than traditional therapy, may still be prohibitive for low-income individuals. It is crucial to critically examine whether the deployment of AI inadvertently creates a two-tiered system, where privileged populations reap the benefits while others are left behind.
The Needs of Underserved Populations
Addressing the needs of underserved populations requires a nuanced understanding of their specific challenges. Rural communities often face a shortage of mental health professionals, making teletherapy and AI-powered self-help tools particularly valuable. However, ensuring reliable internet access and providing training on how to use these technologies are critical prerequisites.
Low-income communities, on the other hand, may struggle with affordability and digital literacy. Subsidized programs and partnerships with community organizations are essential to ensure that AI-driven mental health support is accessible to all, regardless of socioeconomic status.
Moreover, cultural sensitivity is paramount. AI algorithms trained on biased datasets may perpetuate harmful stereotypes or fail to accurately assess the needs of individuals from diverse cultural backgrounds.
Strategies for Equitable Access
Achieving equitable access to AI-driven mental health support requires a multi-pronged approach:
-
Subsidized Programs and Financial Assistance: Governments and non-profit organizations should invest in subsidized programs that provide affordable access to AI-powered mental health tools for low-income individuals and families.
-
Infrastructure Development and Digital Literacy Initiatives: Expanding internet access in rural and underserved areas is crucial. Alongside this, digital literacy programs are needed to empower individuals to effectively utilize AI-driven mental health resources.
-
Culturally Sensitive AI Development: AI algorithms must be trained on diverse and representative datasets to avoid perpetuating biases. Collaboration with cultural experts is essential to ensure that AI interventions are culturally appropriate and sensitive.
-
Community Partnerships and Outreach: Engaging with community organizations and leaders can help build trust and ensure that AI-driven mental health support is effectively tailored to the specific needs of each community.
-
Ethical Guidelines and Regulations: Clear ethical guidelines and regulations are needed to govern the development and deployment of AI in mental healthcare, ensuring that equity and fairness are prioritized.
AI holds immense promise for transforming mental healthcare, but its potential will only be fully realized if access is equitable. By prioritizing the needs of underserved populations, investing in infrastructure and digital literacy, and promoting culturally sensitive AI development, we can ensure that these powerful tools serve as a catalyst for inclusivity, bridging the gap in mental healthcare access and improving the well-being of all. The ethical imperative is clear: innovation must be coupled with a steadfast commitment to equity and social justice.
Autonomy and Control: Safeguarding Patient Choice in the Age of AI
AI’s transformative potential in mental healthcare is undeniable. However, alongside the promise of improved diagnostics and personalized treatment lies a crucial responsibility: ensuring equitable access to these advancements. The question remains: do AI tools exacerbate or alleviate disparities in mental healthcare access? This section delves into how AI impacts patient autonomy, a cornerstone of ethical mental healthcare, and explores methods to safeguard individual choice amidst technological advancements.
The Erosion of Autonomy: A Potential Pitfall
The integration of AI in mental healthcare presents a subtle yet significant risk: the erosion of patient autonomy. As AI systems become more sophisticated in their ability to analyze data and generate recommendations, there is a danger that these systems may unduly influence, or even supplant, the role of the patient in making informed decisions about their own care.
This can manifest in several ways. AI-driven diagnostic tools might lead clinicians to prematurely conclude diagnoses, potentially overlooking crucial subjective experiences reported by the patient. Similarly, AI-powered treatment recommendations could be perceived as prescriptive, diminishing the patient’s sense of agency and their willingness to actively participate in the therapeutic process.
The allure of efficiency and data-driven certainty may inadvertently overshadow the fundamental principle that individuals have the right to self-determination in their mental health journey. The risk is not necessarily malicious, but rather a consequence of over-reliance on technology and a failure to adequately prioritize the patient's voice.
Safeguarding Autonomy: A Multifaceted Approach
Protecting patient autonomy in the age of AI requires a multifaceted approach that encompasses technological design, clinical practice, and ethical guidelines. Several strategies can be employed to mitigate the risk of diminished autonomy.
First and foremost, transparency is paramount. Patients must be fully informed about the role of AI in their treatment, including the capabilities and limitations of the specific AI systems being used. They should understand how the AI arrives at its recommendations, and they should be empowered to question and challenge those recommendations.
The Role of Human Oversight
Secondly, human oversight is indispensable. AI should be viewed as a tool to augment, not replace, the expertise and judgment of mental health professionals. Clinicians must retain the ultimate responsibility for treatment decisions, and they should always prioritize the patient’s individual needs and preferences.
It is imperative that clinicians are adequately trained to critically evaluate AI-generated recommendations, ensuring that these align with the patient's values, goals, and lived experiences.
Empowering Patients Through Education
Furthermore, patient education plays a crucial role. Individuals should be educated about their rights, including the right to refuse AI-driven interventions or seek alternative treatment options. They should also be provided with the resources and support necessary to make informed decisions about their mental healthcare.
Finally, ethical guidelines and regulatory frameworks are essential to ensure that AI is used responsibly and ethically in mental healthcare. These frameworks should address issues such as informed consent, data privacy, and accountability, and they should be regularly updated to reflect the evolving landscape of AI technology.
Balancing AI Recommendations and Human Judgment
The key to successfully integrating AI into mental healthcare lies in finding the right balance between leveraging the capabilities of AI systems and upholding the principles of patient autonomy and human clinical judgment. AI can be a powerful tool for enhancing diagnosis, personalizing treatment, and improving access to care. However, it should never be used in a way that diminishes the patient's sense of agency or undermines the therapeutic relationship.
The ideal scenario is one in which AI serves as a collaborative partner, providing clinicians with valuable insights and supporting them in making informed decisions alongside their patients.
Ultimately, the goal is to create a system that empowers individuals to take control of their mental health journey, while ensuring that they receive the best possible care.
Dehumanization: The Importance of Human Connection in AI-Augmented Therapy
AI’s transformative potential in mental healthcare is undeniable. However, alongside the promise of improved diagnostics and personalized treatment lies a crucial responsibility: ensuring that these technological advancements do not inadvertently erode the very essence of therapeutic care – human connection. The risk of dehumanization, where AI-driven support supplants genuine human interaction, demands careful consideration and proactive mitigation.
The Peril of Replacing Empathy with Algorithms
One of the most significant concerns surrounding the integration of AI in mental healthcare is the potential for algorithmic substitution of human empathy. While AI can process vast amounts of data and identify patterns indicative of mental distress, it fundamentally lacks the capacity for genuine emotional understanding and compassionate responsiveness that characterize effective therapeutic relationships.
This absence of empathy can have profound consequences for patients. Mental healthcare is not merely about identifying and treating symptoms; it is about fostering a sense of trust, validation, and mutual understanding. These elements are cultivated through the nuanced exchange of emotions, non-verbal cues, and shared experiences – aspects of human interaction that AI currently struggles to replicate.
The temptation to rely solely on AI-driven tools for mental health support may arise from their convenience, accessibility, and perceived cost-effectiveness. However, replacing human therapists with algorithms risks creating a sterile and impersonal environment where patients feel more like data points than individuals deserving of care and compassion.
The Crucial Role of the Therapeutic Alliance
The therapeutic alliance, the collaborative relationship between therapist and patient, is a cornerstone of effective mental health treatment. This alliance is built on mutual trust, respect, and a shared understanding of the patient's goals and values. It provides a safe and supportive space for patients to explore their thoughts, feelings, and experiences without judgment.
AI-driven tools can certainly play a role in augmenting and enhancing the therapeutic alliance. For instance, they can assist therapists in gathering data, tracking progress, and providing personalized recommendations. However, it is essential to recognize that AI cannot replace the human therapist as the primary architect of the therapeutic alliance.
The therapeutic alliance thrives on empathy, authenticity, and vulnerability – qualities that are intrinsically human. A therapist's ability to actively listen, reflect, and offer genuine support is critical for fostering a strong therapeutic bond. Over-reliance on AI risks undermining these essential elements, potentially leading to a weaker therapeutic alliance and less favorable outcomes.
Designing AI for Augmentation, Not Substitution
The key to mitigating the risk of dehumanization lies in designing AI systems that complement, rather than substitute, human interaction. AI should be viewed as a tool to empower therapists and enhance their ability to provide effective care, not as a replacement for their expertise and empathy.
This requires a shift in focus from automating therapeutic processes to augmenting human capabilities. AI can be used to:
- Enhance diagnostic accuracy: Assisting therapists in identifying patterns and risk factors that may be missed through traditional methods.
- Personalize treatment plans: Tailoring interventions to individual patient needs and preferences based on data-driven insights.
- Provide access to resources: Connecting patients with relevant information, support groups, and community services.
However, the final decision-making authority should always rest with the human therapist, who can integrate AI-generated insights with their own clinical judgment and understanding of the patient's unique circumstances.
Furthermore, AI systems should be designed with a strong emphasis on transparency and explainability. Patients should be informed about how AI is being used in their treatment and given the opportunity to ask questions and express concerns.
Ultimately, the successful integration of AI in mental healthcare hinges on prioritizing human connection, empathy, and the therapeutic alliance. By designing AI systems that augment, rather than replace, human interaction, we can harness the power of technology to improve mental well-being without sacrificing the essential elements of compassionate care.
AI's Potential to Reshape Mental Health Stigma: A Double-Edged Sword
AI’s transformative potential in mental healthcare is undeniable. However, alongside the promise of improved diagnostics and personalized treatment lies a crucial responsibility: ensuring that these technological advancements do not inadvertently erode the very essence of therapeutic care. The ethical considerations surrounding the use of AI in mental health are multifaceted, demanding careful scrutiny and proactive measures.
One critical area of concern is the potential for AI tools to either reduce or reinforce the stigma associated with mental illness. This section will explore the nuances of this dynamic, focusing on how AI interventions can be designed to promote acceptance and understanding, while also addressing the inherent biases that could perpetuate harmful stereotypes.
The Dual Nature of AI and Stigma
AI presents a paradoxical opportunity in the context of mental health stigma. On one hand, it offers the potential to democratize access to mental health support, particularly for individuals who might otherwise avoid seeking help due to fear of judgment or discrimination.
AI-powered chatbots, for instance, can provide a confidential and non-judgmental space for individuals to express their concerns and receive initial support, potentially breaking down barriers to care.
On the other hand, the use of AI in mental health carries the risk of reinforcing existing stigmas. If AI systems are trained on biased data, they may perpetuate stereotypes and discriminatory practices, leading to inaccurate assessments and inappropriate treatment recommendations.
Designing for Acceptance and Understanding
To harness the potential of AI for reducing stigma, it is essential to design interventions that actively promote acceptance and understanding of mental illness. This requires a human-centered approach that prioritizes empathy, inclusivity, and cultural sensitivity.
AI systems should be developed in collaboration with mental health professionals, patients, and community stakeholders to ensure that they are aligned with ethical principles and best practices.
One key strategy is to use AI to disseminate accurate and evidence-based information about mental illness, challenging common misconceptions and promoting a more nuanced understanding of mental health conditions.
AI can also be used to facilitate peer support networks, connecting individuals with shared experiences and fostering a sense of community.
Addressing Bias in AI-Driven Assessments
A critical challenge in mitigating stigma lies in addressing potential biases in AI-driven assessments. If AI systems are trained on data that reflects societal biases, they may inadvertently perpetuate harmful stereotypes and discriminate against certain groups.
For example, an AI system trained on data that overrepresents certain racial or ethnic groups may misdiagnose or mistreat individuals from other groups.
To address this issue, it is crucial to use diverse and representative datasets in training AI systems. Additionally, algorithms should be carefully designed to minimize bias and ensure fairness across different populations.
Regular audits and evaluations are also essential to identify and correct any biases that may emerge over time. Transparency in AI decision-making can help to build trust and ensure that individuals are treated fairly and equitably.
Responsibility and Accountability: Who is Accountable When AI Makes Mistakes?
AI’s transformative potential in mental healthcare is undeniable. However, alongside the promise of improved diagnostics and personalized treatment lies a crucial responsibility: ensuring that these technological advancements do not inadvertently erode the very essence of therapeutic care. The question of accountability in the event of AI-related errors or harm is paramount, demanding a meticulous examination of legal, ethical, and practical considerations.
The Blame Game: Navigating a Complex Web of Responsibility
When an AI system makes a misdiagnosis, provides harmful advice, or breaches patient privacy, assigning blame becomes a profoundly complex task. Is it the developer who designed the algorithm? The clinician who deployed it? The institution that implemented it? Or is the AI itself somehow responsible?
These questions highlight the limitations of current legal and ethical frameworks, which are often ill-equipped to deal with the nuances of AI-driven decision-making.
The challenge lies in determining the degree to which each party contributed to the adverse outcome and establishing a clear chain of causality.
Legal Frameworks: Untangling the Threads of Liability
Existing legal frameworks, such as product liability laws and medical malpractice statutes, offer a starting point for addressing accountability in AI-related incidents.
However, their applicability to AI systems is often ambiguous. Establishing negligence or causation can be exceptionally difficult when the AI's decision-making process is opaque or when the harm arises from a combination of factors.
Furthermore, the legal status of AI systems themselves remains uncertain. Are they merely tools, like stethoscopes, or are they something more akin to autonomous agents?
The answer to this question will profoundly impact how liability is assigned.
Ethical Imperatives: Beyond Legal Compliance
Beyond legal compliance, ethical considerations demand a more nuanced approach to accountability. Even if a particular action is technically legal, it may still be ethically questionable.
AI developers, clinicians, and institutions have a moral obligation to ensure that AI systems are used responsibly and ethically. This includes taking steps to mitigate bias, protect patient privacy, and ensure transparency in decision-making.
Furthermore, healthcare providers have a responsibility to maintain their own clinical judgment and not blindly rely on AI recommendations.
The human element in mental healthcare, characterized by empathy, compassion, and critical thinking, should not be abdicated to algorithms.
Establishing Clear Lines of Responsibility: A Multi-Stakeholder Approach
To address the challenges of accountability, it is essential to establish clear lines of responsibility for all stakeholders involved in the development and deployment of AI systems in mental healthcare.
AI Developers
Developers must be held accountable for designing algorithms that are free from bias, secure, and transparent. They should also provide adequate documentation and training to ensure that clinicians can use the systems safely and effectively.
Clinicians
Clinicians must be responsible for using AI tools ethically and responsibly, maintaining their clinical judgment, and protecting patient privacy.
They should also be vigilant in identifying and reporting any errors or biases in the AI system.
Institutions
Institutions must be accountable for implementing policies and procedures that promote the responsible use of AI. This includes providing adequate training, establishing clear lines of authority, and monitoring the performance of AI systems.
The Need for New Frameworks: Charting a Course for the Future
Ultimately, addressing the question of accountability in AI-driven mental healthcare will require the development of new legal and ethical frameworks that are specifically tailored to the unique challenges posed by these technologies.
These frameworks must be flexible enough to adapt to the rapidly evolving landscape of AI, while also providing clear guidance on how to assign responsibility in the event of errors or harm.
This is not merely a legal or technical issue; it is a fundamental question of trust and the ethical stewardship of technology in the service of human well-being.
Commodification of Mental Health: Balancing Profit and Patient Needs
AI’s transformative potential in mental healthcare is undeniable. However, alongside the promise of improved diagnostics and personalized treatment lies a crucial responsibility: ensuring that these technological advancements do not inadvertently erode the very essence of ethical and patient-centered care. This section delves into the inherent tensions between commercial interests and patient well-being in the context of AI-driven mental health solutions.
The commodification of mental health services raises critical questions about access, quality, and affordability. It demands a careful examination of how we can align the pursuit of profit with the fundamental ethical obligations to provide equitable and effective care.
The Allure and Peril of Profit-Driven Innovation
The rapid growth of the AI mental health market presents both opportunities and challenges. Venture capital and private investment fuel innovation, driving the development of novel tools and platforms. This influx of capital can lead to more sophisticated and accessible technologies.
However, the imperative to generate profit can also distort priorities. Companies may focus on developing features that maximize revenue rather than those that best serve patient needs. This can lead to a proliferation of superficially appealing but ultimately ineffective or even harmful solutions.
The Impact on Access, Quality, and Affordability
Prioritizing profit can have detrimental consequences for access to mental healthcare. AI solutions may be marketed primarily to affluent populations, exacerbating existing inequalities. The focus on efficiency and scalability can also lead to a decline in the quality of care, as human interaction and personalized attention are replaced by automated systems.
Furthermore, the commodification of mental health can drive up costs, making care unaffordable for many. Subscription models and hidden fees can create financial barriers, preventing those who need help most from accessing it. The pressure to generate revenue can also lead to aggressive marketing tactics and misleading claims about the effectiveness of AI solutions.
Safeguarding Patient Welfare: Strategies for Alignment
Addressing these challenges requires a multi-faceted approach. Regulatory oversight, ethical guidelines, and industry self-regulation are all essential.
-
Robust regulatory frameworks are needed to ensure that AI mental health solutions are safe, effective, and compliant with data privacy regulations.
-
Clear ethical guidelines can help to prevent the exploitation of vulnerable populations and promote transparency in the development and deployment of AI technologies.
-
Industry self-regulation can play a crucial role in fostering a culture of responsible innovation.
Promoting Value-Based Care
A shift towards value-based care models can help align commercial interests with patient needs. By focusing on outcomes rather than volume, these models incentivize providers to deliver high-quality, cost-effective care.
AI can play a vital role in value-based care by enabling more precise diagnoses, personalized treatment plans, and continuous monitoring of patient progress.
Emphasizing Transparency and Data Privacy
Transparency is essential for building trust and ensuring accountability. AI developers should be required to disclose how their algorithms work, what data they use, and how they protect patient privacy.
-
Independent audits and certifications can help to verify these claims and ensure that AI solutions meet rigorous standards.
-
Strong data privacy protections are also crucial to prevent the misuse of sensitive mental health information.
Investing in Publicly Funded Research and Development
Publicly funded research and development can help to ensure that AI mental health solutions are developed in the public interest. By supporting open-source initiatives and academic research, we can create a more level playing field and prevent the dominance of profit-driven companies.
This can help foster a more equitable and accessible mental healthcare landscape for all.
Fostering Collaboration and Dialogue
Ultimately, addressing the ethical challenges of commodification requires collaboration and dialogue among all stakeholders. Patients, clinicians, policymakers, and AI developers must work together to ensure that AI technologies are used to enhance, not undermine, mental well-being. This requires a commitment to transparency, accountability, and a shared vision of ethical and patient-centered care.
The Role of Key Stakeholders: Shaping the Future of AI in Mental Healthcare
AI’s transformative potential in mental healthcare is undeniable. However, alongside the promise of improved diagnostics and personalized treatment lies a crucial responsibility: ensuring that these technological advancements do not inadvertently erode the very essence of ethical and patient-centered care. This necessitates a collaborative approach, one where diverse stakeholders actively shape the trajectory of AI integration, navigating its complexities and mitigating potential risks.
Psychiatrists and Psychologists: Guardians of Clinical Oversight
Psychiatrists and psychologists stand as sentinels of clinical integrity, possessing the expertise to discern the nuanced interplay between AI and patient well-being. Their role extends beyond mere adoption of AI tools; it encompasses critical evaluation, responsible implementation, and continuous monitoring to ensure that AI augments, rather than supplants, human judgment.
They must be actively involved in:
- Assessing the validity and reliability of AI-driven diagnostic tools.
- Interpreting AI-generated insights within the context of a patient's unique history and circumstances.
- Maintaining the therapeutic alliance and providing empathic support.
- Advocating for patient autonomy and informed consent in AI-assisted treatment.
The challenge lies in bridging the gap between technological capabilities and clinical realities, ensuring that AI serves as a valuable adjunct to, and not a replacement for, the essential human elements of mental healthcare.
AI Developers and Engineers: Architects of Ethical Systems
AI developers and engineers wield significant influence in shaping the ethical contours of AI in mental healthcare. Their responsibility extends beyond technical proficiency to encompass a profound understanding of ethical principles and societal values.
This includes:
- Designing algorithms that are fair, transparent, and accountable.
- Implementing robust data privacy and security measures.
- Addressing potential biases in data and algorithms.
- Collaborating with clinicians and ethicists to ensure that AI systems align with clinical best practices.
The onus is on developers to create AI tools that are not only technologically advanced but also ethically sound, fostering trust and promoting equitable access to mental healthcare.
Data Scientists: Stewards of Privacy and Integrity
Data scientists occupy a critical juncture, tasked with harnessing the power of data while safeguarding patient privacy and ethical considerations. Their focus is not solely on extracting insights but also on ensuring that data is handled responsibly and ethically.
This involves:
- Implementing rigorous anonymization and pseudonymization techniques.
- Adhering to data protection regulations such as GDPR and HIPAA.
- Addressing potential biases in data collection and analysis.
- Promoting transparency and explainability in AI models.
Data scientists must act as stewards of data integrity, upholding the highest ethical standards and ensuring that patient privacy is paramount.
Ethicists: Navigating the Moral Compass
Ethicists serve as vital guides, providing critical analysis and recommendations on the responsible deployment of AI in mental healthcare. Their expertise lies in identifying potential ethical dilemmas, assessing their implications, and formulating ethical frameworks to guide decision-making.
They are instrumental in:
- Developing ethical guidelines and standards for AI in mental healthcare.
- Facilitating dialogue and deliberation among stakeholders.
- Providing ethical consultations on complex cases involving AI.
- Advocating for policies that promote ethical AI development and deployment.
Policymakers and Regulators: Establishing Guardrails and Oversight
Policymakers and regulators play a crucial role in establishing the legal and regulatory framework for AI in mental healthcare. Their responsibilities include:
- Creating policies that promote innovation while safeguarding patient rights and safety.
- Developing regulatory mechanisms to ensure that AI systems are safe, effective, and ethical.
- Addressing issues of liability and accountability in cases of AI-related harm.
- Promoting equitable access to AI-driven mental health services.
Effective policy and regulation are essential to fostering a responsible and trustworthy AI ecosystem in mental healthcare.
Mental Health Advocates: Amplifying the Patient Voice
Mental health advocates play a pivotal role in ensuring that the voices and needs of patients are central to the AI discourse. They advocate for:
- Patient autonomy and informed consent.
- Equitable access to AI-driven mental health services.
- The reduction of stigma associated with mental illness.
- The development of AI systems that are sensitive to the needs of diverse populations.
By amplifying the patient voice, advocates ensure that AI serves the best interests of those it is intended to help.
Patients and Users: The End-Users' Experience
Patients and users are the ultimate recipients of AI-driven mental health tools, and their experiences are paramount. Their feedback is crucial for:
- Evaluating the usability and effectiveness of AI systems.
- Identifying potential biases and unintended consequences.
- Ensuring that AI systems meet their needs and preferences.
- Promoting trust and acceptance of AI in mental healthcare.
Involving patients and users in the design and evaluation of AI systems is essential for creating tools that are truly patient-centered.
Researchers: Illuminating the Path Forward
Researchers play a crucial role in advancing our understanding of the impact of AI on mental healthcare. Through rigorous scientific inquiry, they can:
- Evaluate the effectiveness of AI interventions.
- Identify potential risks and benefits.
- Develop new methods for mitigating bias and enhancing transparency.
- Inform policy and practice through evidence-based findings.
Research is essential for guiding the responsible development and deployment of AI in mental healthcare, ensuring that it is grounded in scientific evidence and ethical principles.
Organizations Leading the Way: Setting Standards for Ethical AI in Mental Healthcare
AI’s transformative potential in mental healthcare is undeniable. However, alongside the promise of improved diagnostics and personalized treatment lies a crucial responsibility: ensuring that these technological advancements do not inadvertently erode the very essence of ethical patient care. This requires the concerted efforts of numerous organizations dedicated to setting and upholding standards for ethical AI implementation.
From international bodies to grassroots advocacy groups, these entities play a pivotal role in shaping the future of AI in mental healthcare. By promoting responsible development, deployment, and oversight, they strive to maximize the benefits of AI while minimizing potential risks.
The World Health Organization (WHO): Global Guidance on AI in Healthcare
The World Health Organization has recognized the profound implications of AI for global health. The WHO provides guidance and recommendations to member states on the ethical and responsible use of AI in healthcare, including mental health.
Their focus encompasses ensuring equitable access to AI-driven solutions, protecting patient privacy, and promoting transparency in AI algorithms. The WHO emphasizes the need for a human-centered approach to AI, where technology serves to augment, rather than replace, human clinicians and caregivers.
The WHO also underscores the importance of international collaboration in developing ethical frameworks and standards for AI in healthcare, facilitating the sharing of best practices and mitigating potential risks across borders.
National Institute of Mental Health (NIMH): Advancing Research and Ethical Considerations
The National Institute of Mental Health plays a critical role in funding and conducting research on mental illness. NIMH-supported research aims to improve our understanding of mental disorders and develop innovative treatments, including those that leverage AI.
Recognizing the ethical implications of AI in mental health, NIMH also supports research that explores the potential risks and benefits of AI-driven interventions. This includes studies on algorithmic bias, data privacy, and the impact of AI on the therapeutic relationship.
By investing in research that addresses these ethical considerations, NIMH seeks to ensure that AI technologies are developed and deployed in a responsible and equitable manner, promoting positive outcomes for individuals with mental health conditions.
AI Ethics Organizations: Championing Responsible AI Development
A multitude of organizations are dedicated to promoting ethical AI development and deployment across various sectors, including healthcare. These organizations bring together experts from diverse fields, such as computer science, ethics, law, and social sciences.
They work to develop ethical frameworks, guidelines, and best practices for AI development, emphasizing principles such as fairness, transparency, accountability, and respect for human autonomy.
These AI ethics organizations often provide resources and training to help developers and organizations implement ethical AI practices, fostering a culture of responsibility and promoting the development of AI systems that align with societal values.
Examples include the Partnership on AI, the AI Ethics Lab, and the IEEE Global Initiative on Ethics of Autonomous and Intelligent Systems.
Technology Companies: Balancing Innovation and Ethics
Technology companies are at the forefront of developing and deploying AI technologies in mental healthcare. While driven by innovation and market opportunities, these companies also face growing pressure to address the ethical implications of their products.
Some technology companies have established internal ethics boards and developed ethical guidelines for AI development. They are investing in research to mitigate algorithmic bias, protect patient privacy, and enhance the transparency of AI systems.
Furthermore, some tech companies are collaborating with researchers and ethicists to develop best practices for responsible AI deployment, ensuring that their technologies are used in a way that promotes ethical patient care and minimizes potential harms.
However, vigilance is needed to ensure that profit motives do not overshadow ethical considerations, and that transparency and accountability are prioritized in the development and deployment of AI mental health solutions.
Government Agencies Regulating Healthcare: Ensuring Compliance and Patient Safety
Government agencies play a crucial role in regulating healthcare and ensuring patient safety. As AI technologies become more prevalent in mental healthcare, these agencies are tasked with adapting existing regulations and developing new policies to address the unique challenges posed by AI.
For example, agencies like the Food and Drug Administration (FDA) may regulate AI-driven diagnostic tools or therapeutic interventions, requiring them to meet certain standards of safety and efficacy before they can be marketed and used.
Furthermore, government agencies are responsible for enforcing data privacy regulations, such as HIPAA in the United States and GDPR in Europe, ensuring that sensitive patient data is protected when used in AI systems.
These regulatory efforts are essential for establishing clear guidelines for AI developers, clinicians, and institutions, promoting responsible AI implementation and safeguarding patient rights.
Patient Advocacy Groups: Amplifying Patient Voices and Promoting Ethical AI
Patient advocacy groups play a vital role in representing the interests of individuals with mental health conditions. These groups advocate for policies and practices that promote access to quality care, protect patient rights, and reduce stigma associated with mental illness.
As AI technologies become increasingly integrated into mental healthcare, patient advocacy groups are working to ensure that these technologies are developed and deployed in a way that aligns with patient needs and values.
They advocate for transparency in AI algorithms, promote patient autonomy in decision-making, and work to ensure that AI-driven interventions are culturally sensitive and accessible to all individuals, regardless of their background or circumstances.
By amplifying patient voices and promoting ethical AI implementation, patient advocacy groups help shape a future where AI enhances, rather than undermines, the well-being of individuals with mental health conditions.
Contextual Factors: AI on Online Platforms and Social Media - A New Frontier for Ethical Concerns
AI’s transformative potential in mental healthcare is undeniable. However, alongside the promise of improved diagnostics and personalized treatment lies a crucial responsibility: ensuring that these technological advancements do not inadvertently erode the very essence of ethical practice. Online platforms and social media, with their pervasive reach and unique dynamics, present a particularly complex landscape where the deployment of AI in mental health demands careful scrutiny.
The Dual-Edged Sword of AI in Online Mental Health
Social media platforms, once primarily domains for social interaction, are increasingly becoming spaces for mental health support. AI-powered tools, from sentiment analysis algorithms detecting users at risk to chatbots offering immediate assistance, are being integrated into these platforms. This integration, while offering the potential for scalable and accessible support, introduces a host of ethical challenges that require careful consideration.
One of the most pressing concerns revolves around the blurring of boundaries between public and private life. Social media, by its very nature, encourages a degree of self-disclosure. However, when users interact with AI-driven mental health tools on these platforms, the sensitivity of the information shared is significantly heightened.
The potential for data breaches and misuse becomes a critical consideration.
Data Security and Privacy: A Precarious Balance
The collection, storage, and analysis of user data by AI systems on social media raise profound privacy concerns. Mental health information is inherently sensitive, and its exposure could lead to stigmatization, discrimination, or even exploitation.
Platforms must implement robust security measures to safeguard user data from unauthorized access and ensure compliance with relevant data protection regulations, such as GDPR and HIPAA (where applicable).
Furthermore, users need to be fully informed about how their data is being used, who has access to it, and the potential risks involved.
The Challenge of Misinformation and Malicious Use
The open and often unregulated nature of social media also presents the risk of misinformation and malicious use of AI-driven mental health tools. AI chatbots, for instance, could be exploited to spread harmful advice or engage in manipulative tactics.
Similarly, sentiment analysis algorithms could be used to target vulnerable users with personalized advertisements or propaganda.
Platforms must develop strategies for detecting and mitigating these risks, including implementing content moderation policies, fact-checking mechanisms, and user education programs.
The Erosion of Trust and Therapeutic Relationship
Traditional mental healthcare relies heavily on the therapeutic relationship, a bond of trust and empathy between the patient and the clinician. The introduction of AI into this equation raises concerns about the potential for dehumanization and the erosion of this vital connection.
While AI can offer valuable support, it cannot replicate the nuance, empathy, and human understanding that are essential for effective mental healthcare.
Over-reliance on AI could lead to a diminishment of human interaction, potentially exacerbating feelings of isolation and alienation, especially among vulnerable populations.
The Need for Human Oversight and Intervention
It is crucial to recognize that AI should be viewed as a tool to augment, not replace, human clinicians.
Human oversight is essential to ensure that AI systems are used ethically and effectively.
Clinicians must be involved in the design, implementation, and monitoring of AI-driven mental health tools on social media platforms.
They should also be available to provide support and intervention when AI systems encounter complex or sensitive situations.
Navigating the Future: A Call for Responsible Innovation
The integration of AI into mental health support on online platforms and social media holds immense potential, but it also carries significant ethical responsibilities. A multi-faceted approach is needed that involves collaboration between technology developers, mental health professionals, policymakers, and the public.
This approach must prioritize data security, transparency, user autonomy, and the preservation of the therapeutic relationship. By embracing these principles, we can harness the power of AI to improve mental health outcomes while safeguarding the well-being and dignity of individuals in the digital age.
Impact of Technology: A Closer Look at Chatbots, Apps, and Teletherapy Platforms
AI’s transformative potential in mental healthcare is undeniable. However, alongside the promise of improved diagnostics and personalized treatment lies a crucial responsibility: ensuring that these technological advancements do not inadvertently erode fundamental ethical principles. This section delves into the specific ethical implications arising from the use of AI-driven technologies, focusing on chatbots, mobile applications, and teletherapy platforms. We evaluate the benefits and risks associated with each, offering insights into best practices for responsible implementation.
The Ethical Tightrope of AI-Powered Chatbots
AI-powered chatbots have emerged as a readily accessible and scalable solution for delivering mental health support. These bots offer instant responses, 24/7 availability, and a degree of anonymity that can encourage individuals to seek help. However, their deployment introduces a complex web of ethical considerations.
Deception and Transparency
A primary concern revolves around transparency and the potential for deception. Users must be unequivocally informed that they are interacting with an AI and not a human therapist. Failure to do so can undermine trust and compromise the therapeutic relationship, however nascent. The illusion of empathy, while seemingly benign, can be particularly problematic if users misinterpret the AI’s responses as genuine emotional understanding.
Data Security and Privacy Risks
Chatbots collect vast amounts of sensitive personal data, making them attractive targets for cyberattacks. Robust data encryption, stringent access controls, and compliance with regulations such as HIPAA and GDPR are paramount to safeguarding user privacy.
Furthermore, data sharing practices must be transparently disclosed and users must have control over their data. Data collected must be strictly limited to only what is crucial and necessary.
Competency and Scope Limitations
AI chatbots are not substitutes for qualified mental health professionals. Their capabilities are limited, and they are not equipped to handle complex or crisis situations. It is imperative that chatbots are programmed to recognize their limitations and to seamlessly escalate users to human support when necessary. The possibility of incorrect or harmful advice dispensed by AI is also a serious ethical consideration.
Mobile Applications: Navigating a Landscape of Variable Quality
Mobile applications designed to provide mental health support have proliferated in recent years, offering a range of features from mood tracking to guided meditations. While these apps hold the potential to empower individuals and improve access to care, they also present unique ethical challenges.
The Wild West of Unregulated Innovation
The mental health app market is largely unregulated, leading to a proliferation of apps with questionable efficacy and varying degrees of ethical oversight. Many apps lack scientific validation. This leaves users vulnerable to ineffective or even harmful interventions. Independent evaluation and certification processes are needed to ensure quality and safety.
Data Monetization and User Exploitation
Many mental health apps rely on data collection and monetization as a primary revenue stream. This raises concerns about the potential for user data to be exploited for commercial gain, particularly if data is shared with third-party advertisers or data brokers without explicit consent. The ethics of profiting from vulnerable individuals’ mental health data are highly dubious.
The Risk of Digital Divide and Inequity
While mobile apps can improve access to mental health support, they can also exacerbate existing inequalities. Individuals from low-income backgrounds or those lacking digital literacy may be excluded from accessing these resources. Efforts must be made to ensure that digital mental health solutions are accessible and equitable.
Teletherapy Platforms: Balancing Access and Quality
Teletherapy platforms have emerged as a vital tool for delivering mental health services remotely, particularly in underserved areas or during times of crisis. However, the use of technology in therapy also raises ethical considerations that must be addressed to ensure patient well-being.
Maintaining Confidentiality in the Digital Realm
Ensuring the confidentiality and security of teletherapy sessions is crucial. Platforms must employ robust encryption protocols and adhere to HIPAA regulations to protect patient data from unauthorized access. Therapists must also take precautions to ensure privacy on their end, such as using secure internet connections and conducting sessions in private locations.
Licensing and Cross-Jurisdictional Practice
The provision of teletherapy across state or national borders raises complex licensing and regulatory issues. Therapists must be licensed in the jurisdiction where the patient is located. Platforms must ensure that therapists are properly credentialed and comply with all applicable regulations.
The Importance of the Therapeutic Alliance
The therapeutic alliance, the relationship between the therapist and the patient, is a critical predictor of treatment success. Teletherapy can present challenges to building and maintaining this alliance. Therapists must adapt their communication skills to effectively engage with patients remotely. They need to create a safe and supportive online environment.
Accessibility Considerations
Teletherapy platforms need to be user-friendly and accessible to individuals with disabilities. Platforms must comply with accessibility standards such as WCAG to ensure that users with visual, auditory, or motor impairments can fully participate in therapy. This includes providing captioning for video sessions, screen reader compatibility, and alternative input methods.
Video: Social Implications: AI Mental Health - Risks?
FAQs: Social Implications of AI Mental Health - Risks
Can AI mental health tools worsen existing social inequalities?
Yes. Bias in training data can lead to AI tools that are less effective, or even harmful, for marginalized groups. This reinforces existing social inequalities in access to quality mental healthcare.
How might AI mental health apps affect human connection?
Over-reliance on AI for support could reduce real-world social interactions and empathy. This potential decline in genuine human connection poses a significant social implication.
What are the data privacy risks of using AI for mental health?
AI systems collect and analyze sensitive personal information. Data breaches or misuse could expose individuals to stigma, discrimination, or exploitation, raising crucial social implications regarding data security.
Could reliance on AI mental health tools reduce trust in human professionals?
Potentially. If people perceive AI as a cheaper or more convenient alternative, trust in therapists and mental health professionals might decrease, altering the dynamics and future of social attitudes towards mental healthcare.
So, as we navigate this brave new world of AI and mental health, it's crucial to keep these potential pitfalls in mind. The benefits are undeniable, but a critical and cautious approach is vital. Ultimately, understanding the social implications of AI in mental health will help us ensure this technology empowers, rather than endangers, our well-being.